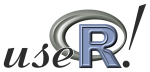 |
Tutorial: Exploratory Data Analysis
|
Julie Josse, Agrocampus Rennes, Rennes Centre for Higher Education and Research in Agronomy, Rennes Cedex, France.
François Husson, Agrocampus Rennes, Rennes Centre for Higher Education and Research in Agronomy, Rennes Cedex, France.
Sébastien Lê, Agrocampus Rennes, Rennes Centre for Higher Education and Research in Agronomy, Rennes Cedex, France.
Motivation
Nowadays, researchers have to handle complex data, hence the need to sum them up and to visualize the information in a proper and convenient way.
This course gives a detailed overview of the classical statistical methods for data mining and exploratory data analysis: Principal Components Analysis, Correspondence Analysis, Multiple Correspondence Analysis.
We will stress on the importance to enrich the interpretation of the outputs by adding supplementary information and using specific indicators.
We illustrate these different methods through data sets providing from many fields such as genomic data, ecological data, sensory data.
Outline
- Introduction to multivariate exploratory data analysis:
- Main objectives of these methods: reducing the dimensionality of the data sets, sum up the information, individuals and variables typology, ...
- b. Type of variables used: continuous or categorical variables and possibly both
- Exploratory analysis of continuous data by the use of Principal Components Analysis:
- Simultaneous interpretation of the individuals graphs and the variables ones using the transition formulae
- How to introduce supplementary information such as supplementary individuals, supplementary continuous or categorical variables? Supplementary information does not participate to the construction of the analysis but is a tool to facilitate the interpretation of the analysis. How to describe quickly the dimensions?
- Exploratory analysis of categorical data (such as survey data)
- From a one-dimensional point of view, automatic characterization of the levels of each categorical variable (with continuous and categorical variables)
- From a multi-dimensional point of view with Correspondence Analysis (for two variables) and Multiple Correspondence Analysis (for more than two variables)
The different methods will be illustrated with numerous examples and we will use one or more packages such as FactoMineR.
Background knowledge
No prior knowledge is required
Intended audience
Teachers in data mining and data analysis, researchers in applied field, statisticians whose topic of interest is multivariate analysis
Workshop materials
More information will be available (notes, scripts, and data sets) at our website (http://factominer.free.fr)